- Tomorrow Bytes
- Posts
- Beyond Bigger: AI's Pivot From Size to Smarts
Beyond Bigger: AI's Pivot From Size to Smarts
Tomorrow Bytes #2427
AI's relentless march continues, reshaping industries from finance to design. Goldman Sachs unveils a centralized AI platform, boosting developer efficiency by 20%, while Figma introduces AI features to streamline designers' workflows. The AI gold rush has propelled Nvidia to unprecedented valuations, yet a $600 billion gap looms between investments and revenue. As language models plateau, the industry pivots to smarter, data-driven approaches. This week, we dive into the evolving AI landscape, exploring how businesses navigate the paradox of speculative frenzies and tangible value creation. From AI-powered Olympics coverage to the rise of machine critics, we unpack the transformative potential and challenges facing AI adoption across sectors.

🔦 Spotlight Signals
Character.AI introduces a voice calling feature for its AI characters, expanding beyond text interactions, with over 3 million users making more than 20 million calls during the testing phase.
Figma introduces AI features, including visual search, auto-generated content, and quick prototyping. It aims to streamline designers' workflows and boost creativity while allowing organizations to opt out of AI training using their data until August 15, 2024.
NBC will use an AI-generated version of sportscaster Al Michaels' voice to deliver personalized daily recaps of the Summer Olympics. It will offer subscribers 10-minute highlight packages that can be packaged in about 7 million different ways based on 5,000 hours of live coverage.
OpenAI has acquired Multi, a five-person startup specializing in screen sharing and collaboration technology, to bolster its ChatGPT desktop app team. This is OpenAI’s third known acquisition as it expands its AI capabilities.
Apple declined Meta's offer to integrate its Llama chatbot into the iPhone, highlighting the tech giant's cautious approach to AI partnerships as it develops its own solutions.
Toys "R" Us debuted a 66-second promotional film at the 2024 Cannes Lions Festival, created almost entirely using OpenAI's unreleased Sora text-to-video tool, potentially signaling a shift in advertising production methods.
TIME magazine announces strategic partnerships with OpenAI and ElevenLabs. OpenAI will be granted access to its 101-year archive for AI product enhancement, and ElevenLabs will implement its Audio Native technology to provide automated voiceovers on TIME.com. Both initiatives aim to expand access to trusted journalism through innovative AI-driven approaches.
Goldman Sachs is rolling out its first generative AI tool for code generation to thousands of developers. The tool leverages a centralized internal platform that incorporates multiple AI models and has reportedly increased developer efficiency by 20%.
Generative AI is poised to revolutionize accounting. It will address industry challenges like an aging workforce and labor shortages while automating key tasks such as data collection, research, report generation, and client advisory services.
Colorado pioneers AI regulation with a comprehensive law set to take effect in 2026 aimed at preventing bias and discrimination in consequential decision-making across key industries.

💼 Business Bytes
AI's $600 Billion Gamble: Profit or Pipe Dream?
The AI gold rush has propelled Nvidia to the top of global valuations and filled OpenAI's coffers. Yet a chasm yawns between colossal infrastructure investments and realized revenue. This "$600B question" looms over the industry, challenging assumptions about AI's economic potential.
GPU supply shortages have eased, but cloud giants continue stockpiling chips. Nvidia's upcoming B100 promises another leap in performance, likely triggering fresh demand spikes and supply crunches. These cycles of scarcity and abundance ripple through the entire tech ecosystem, shaping investment strategies and market dynamics.
For businesses, the AI landscape presents a paradox. While speculative frenzies echo past tech bubbles, AI's potential for value creation remains immense. Success will hinge on prioritizing end-user benefits and long-term innovation over short-term gains. As GPU computing commoditizes, nimble startups may find new opportunities amidst the turbulence.
[Dive In]
Tomorrow Bytes’ Take…
Evolving Market Dynamics: The AI ecosystem is rapidly changing, with Nvidia becoming the most valuable company globally and OpenAI holding a significant share of AI revenue.
Infrastructural Investment vs. Revenue Realization: Despite massive investments in AI infrastructure, a substantial gap exists between expected and actual revenue, now termed the "$600B question."
GPU Supply and Demand Trends: The GPU supply shortage has subsided, but major cloud providers' stockpiling indicates potential future adjustments in demand.
Economic Implications of AI Advancements: New advancements like Nvidia's B100 chip will likely drive demand and lead to another supply shortage, impacting market dynamics and investment strategies.
Long-term Viability and Value Creation: Despite speculative investment frenzies, AI can potentially create significant economic value, especially for those focusing on end-user value and long-term innovation.
Commoditization of GPU Computing: GPU computing is becoming commoditized, leading to reduced pricing power for providers and potential market saturation.
Depreciation of Technology Investments: Rapid advancements in AI technology, such as next-gen chips, will lead to faster depreciation of current investments, challenging the long-term value of existing infrastructure.
Speculative Investment Risks: Historical parallels with railroads suggest high capital incineration rates during speculative technology waves, with more losers than winners.
Strategic Investment and Innovation Opportunities: Declining costs for GPU computing will foster innovation and benefit startups despite challenges for investors.
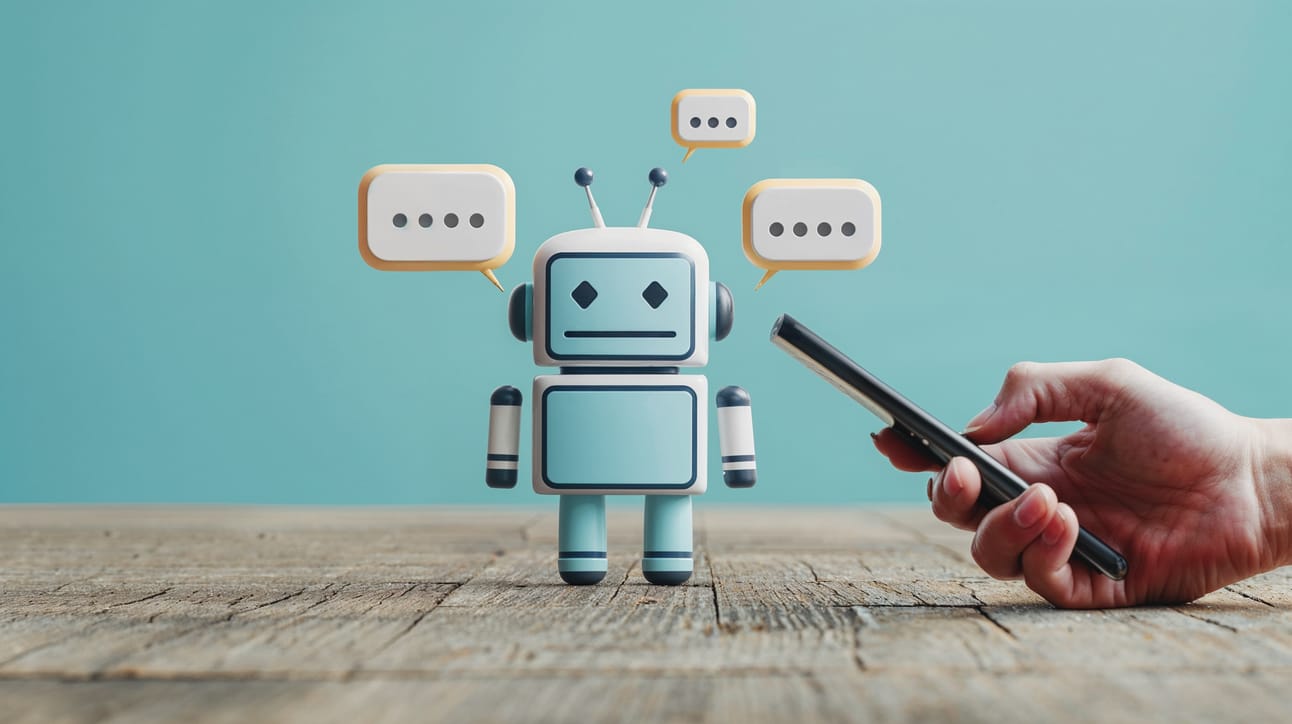
☕️ Personal Productivity
The AI Assistant Mirage: Promise Meets Reality
AI work assistants arrived with grand promises of effortless productivity. Reality has proven more complex. Enterprises grapple with data quality issues, immature technology, and the need for extensive user training. These challenges transform the implementation of tools like Copilot and Gemini from a simple plug-and-play solution into a significant organizational undertaking.
Data emerges as the critical battleground. Inconsistent, duplicated, outdated information plagues many companies, hobbling AI performance. This forces a reckoning with long-neglected data management practices. Vendors scramble to provide solutions, but the onus remains on businesses to clean house.
Despite frustrations, optimism persists. CIOs see these hurdles as growing pains on the path to transformative productivity gains. Companies that master the art of data curation and user education will reap outsized rewards. For others, expensive AI assistants risk becoming shelfware – a cautionary tale of technology outpacing organizational readiness.
[Dive In]
Tomorrow Bytes’ Take…
Enterprise Adoption Challenges: Implementing AI work assistants like Copilot and Gemini requires significant internal effort, contrary to initial expectations of ease.
Data Quality Issues: Accurate and up-to-date data is critical for the effectiveness of AI tools. Enterprises face challenges with inconsistent, duplicated, and outdated data, which hinders AI performance.
Maturity of AI Tools: Current AI tools still have limitations, often providing inaccurate or outdated responses due to immature technology and reliance on suboptimal data.
Importance of Data Management: Implementing AI work assistants requires rigorous data cleaning, validation, and management to ensure reliable outputs.
Vendor Acknowledgment and Response: Vendors like Microsoft and Google know these challenges and are developing tools and strategies, such as Copilot Studio, to help enterprises direct AI tools to authoritative data sources.
User Training and Prompting: Effective use of AI assistants requires users to understand the importance of providing context and learning the art of prompting to get accurate answers.
Long-term Optimism: Despite current hurdles, CIOs and technology leaders strongly believe that AI work assistants will eventually deliver on their productivity promises.
Catalyst for Data Management Improvements: The desire to leverage AI work assistants pushes organizations to prioritize data management, leading to better data practices.
Cost Considerations: AI tools come with a significant price tag, emphasizing the need for organizations to ensure they derive maximum value from these investments.

🎮 Platform Plays
Anthropic's AI Revolution: Redefining Team Collaboration
Anthropic's Claude unveils a new frontier in AI-assisted teamwork. Projects and Artifacts transform Claude from a mere chatbot into a centralized hub of organizational knowledge and creativity. The 200,000-token context window – equivalent to a 500-page book – allows Claude to digest and leverage vast amounts of company-specific information, addressing the perennial 'cold start' problem plaguing AI adoption.
This leap forward promises to reshape how teams create, collaborate, and make decisions. Users can now generate and edit content alongside AI conversations, streamlining everything from code development to marketing copy. Granular permissions ensure data security while fostering collaboration. Early adopters report completing tasks up to five times faster, hinting at the technology's transformative potential.
Yet Anthropic's vision extends beyond mere efficiency gains. By emphasizing human augmentation rather than replacement, they position Claude as a tool to enhance, not supplant, human capabilities. As integrations with popular business tools expand, we may witness the birth of a new paradigm in knowledge work – one where AI becomes an indispensable member of every team.
[Dive In]
Tomorrow Bytes’ Take…
Revolutionizing AI Teamwork: Anthropic's new features, Projects, and Artifacts significantly enhance AI-assisted collaboration and productivity by centralizing knowledge and AI interactions in one accessible space.
Large Context Window: Claude’s 200,000-token context window allows it to process and understand vast amounts of organization-specific information, improving its ability to provide tailored assistance and address the ‘cold start’ problem in AI.
Enhanced Creative Processes: Artifacts enable users to generate and edit content alongside their conversations with Claude, streamlining creative tasks and improving efficiency in code, design, and content creation.
Advanced Sharing Capabilities: Granular permission settings and advanced sharing features enhance collaboration while maintaining data security and protecting sensitive information.
Future Integrations: Anthropic plans to integrate Claude with popular applications and tools, suggesting a continued focus on seamless AI integration into existing business processes.
Human-AI Collaboration: Anthropic emphasizes enhancing human capabilities with AI rather than replacing them, positioning Claude as a tool for augmenting human work.
Customized AI Outputs: Users can define custom instructions for each Project, tailoring Claude’s responses to specific roles, industries, or desired tones, enhancing relevance and effectiveness.
Improved AI-Assisted Decision Making: Projects and Artifacts help teams with strategic decision-making by grounding AI outputs in internal knowledge and facilitating expert assistance across various tasks.
Significant Productivity Gains: Enterprises using Claude report substantial productivity improvements, with tasks completed up to 5x faster, demonstrating the practical benefits of AI-assisted collaboration.

🤖 Model Marvels
The AI Arms Race Hits a Wall
Hugging Face's revamped Open LLM Leaderboard signals a pivotal shift in AI evaluation. Introducing more rigorous metrics - from multi-turn dialogues to non-English tests - reflects a growing realization: breakthrough improvements in large language models have plateaued. This new landscape demands more nuanced ways to distinguish top performers.
The leaderboard's expansion addresses a critical need for global representation in AI capabilities. Incorporating non-English evaluations acknowledges the diverse requirements of an international user base. Complementary efforts like the LMSYS Chatbot Arena, focusing on real-world interactions, further enrich our understanding of AI performance.
These developments carry profound implications for the AI industry. Enterprise decision-makers now have more sophisticated tools to guide their adoption strategies. The open-source community faces both a challenge and an opportunity to innovate within tighter constraints. As AI evolves, these refined evaluation methods will prove crucial in navigating an increasingly complex technological landscape.
[Dive In]
Tomorrow Bytes’ Take…
Enhanced AI Model Evaluation: Hugging Face’s revamped Open LLM Leaderboard introduces more challenging datasets, multi-turn dialogue evaluations, non-English language tests, and assessments for instruction-following and few-shot learning, providing a comprehensive and nuanced evaluation of AI models.
Plateau in Performance Gains: The AI community is experiencing a slowdown in breakthrough improvements for large language models, highlighting the need for more rigorous evaluation metrics to distinguish top-performing models.
Global Representation: Including non-English language evaluations ensures a broader representation of global AI capabilities, addressing the diverse needs of an international user base.
Complementary Evaluation Approaches: The parallel efforts by the LMSYS Chatbot Arena, which emphasizes real-world, dynamic evaluation through direct user interactions, complement the structured benchmarks of the Open LLM Leaderboard, offering a holistic view of AI performance.
Informed Enterprise Decisions: Enhanced evaluation tools provide enterprise decision-makers with a nuanced view of AI capabilities, which is essential for informed AI adoption and integration strategies.
Fostering Innovation: By offering more sophisticated evaluation methods, these tools foster healthy competition and innovation within the open-source AI community, driving the development of more advanced AI models.
Future of AI Evaluation: As AI models continue to evolve, advancements in evaluation methods, such as those seen in the Open LLM Leaderboard and Chatbot Arena, will be crucial for navigating the complexities of the AI landscape.

🎓 Research Revelations
AI's Self-Scrutiny: The Rise of Machine Critics
CriticGPT heralds a new era in AI development, where machines critique their kind. This GPT-4-based model outperforms human trainers in identifying ChatGPT's errors 60% of the time, revolutionizing the reinforcement learning process. CriticGPT augments human judgment to address the growing challenge of subtle mistakes in increasingly sophisticated AI outputs.
The implications stretch beyond mere error detection. CriticGPT's integration into the RLHF pipeline promises to refine AI alignment, ensuring outputs match human intent and expectations better. Trainers' preference for CriticGPT's critiques over ChatGPT's own – especially for naturally occurring bugs – underscores its potential to elevate the quality of AI-generated content across industries.
This development marks a significant shift in human-AI collaboration. As AI systems grow more complex, tools like CriticGPT become essential for practical evaluation and improvement. The ability to balance precision and recall in critiques opens new avenues for customizing AI behavior to specific needs. In essence, CriticGPT represents an improvement in AI technology and a fundamental change in how we approach AI development and quality control.
[Dive In]
Tomorrow Bytes’ Take…
Enhanced Error Detection: CriticGPT, based on GPT-4, helps human trainers identify errors in ChatGPT's responses, outperforming unassisted human trainers 60% of the time.
Improved RLHF Process: Integrating CriticGPT-like models into the RLHF (Reinforcement Learning from Human Feedback) labeling pipeline enhances the evaluation and alignment of advanced AI systems, addressing the challenge of increasingly subtle mistakes in AI outputs.
Human-AI Collaboration: Combining human and AI efforts results in more comprehensive critiques and fewer hallucinated bugs, demonstrating the potential of AI to augment human capabilities in evaluating AI responses.
Preferred Critiques: Trainers prefer CriticGPT's critiques over ChatGPT's in 63% of cases, especially for naturally occurring bugs, due to fewer nitpicks and hallucinations.
Customizable Critique Precision: By using a test-time search against the critique reward model, CriticGPT can balance precision and recall, generating longer and more comprehensive critiques tailored to RLHF needs.
Training Methodology: CriticGPT was trained with RLHF using many inputs containing manually inserted mistakes, enabling it to provide detailed and accurate critiques.
Future Implications: Developing and scaling CriticGPT-like models will be crucial for aligning increasingly complex AI systems, ensuring they can be evaluated and improved.

🚧 Responsible Reflections
The Great AI Scale-Back: Bigger Isn't Always Better
The AI industry's obsession with ever-larger language models is hitting a wall. Contrary to popular belief, simply scaling up model size no longer guarantees proportional gains in capability. This reality check is forcing a seismic shift in AI development strategies, with far-reaching implications for businesses and researchers.
Data, not size, has become the new bottleneck. As models like Llama 3 gobble up trillions of tokens, high-quality training material grows scarce. Synthetic data offers a partial solution but can't fully replace the nuanced complexity of human-generated content. This scarcity drives a pivot towards smaller, more intensively trained models that balance performance with economic viability.
This recalibration of the AI landscape favors innovation over brute force. Companies are abandoning the pursuit of headline-grabbing massive models, recognizing them as unsustainable vanity projects. The future of AI lies in smarter training methods, improved data curation, and models tailored for specific tasks. As the industry matures, the winners will be those who can extract maximum capability from minimal resources, not those with the biggest computational hammer.
[Dive In]
Tomorrow Bytes’ Take…
Misunderstood Scaling Laws: The belief that continuous scaling of language models will yield better AI capabilities indefinitely is a misinterpretation. Scaling laws primarily reflect improvements in perplexity, not emergent abilities, and there’s no empirical evidence that scaling alone will lead to AGI.
Data Limitations: High-quality training data is becoming a bottleneck. The AI industry is nearing the limits of readily available, high-quality data sources, making further scaling more challenging and expensive.
Synthetic Data Constraints: Synthetic data is useful for filling specific gaps but cannot replace high-quality human data for training LLMs, limiting its potential for continued scaling.
Shift in Development Focus: AI developers are moving towards smaller models with longer training periods to achieve desired performance levels, balancing training and inference costs.
Market Trends and Business Decisions: Building larger models is no longer seen as a wise business move due to the high costs and diminishing returns. Instead, there’s a focus on optimizing existing capabilities and developing more cost-effective models.
System 2 --> System 1 Distillation: Self-play and similar strategies are effective in specific, contained environments like games but are not broadly applicable to more open-ended tasks.
Future Research Directions: Future advancements may rely more on improving the quality of training data and finding innovative ways to enhance model performance without relying solely on scaling.
We hope our insights sparked your curiosity. If you enjoyed this journey, please share it with friends and fellow AI enthusiasts.